- Published on
OpenAI Sora FQA
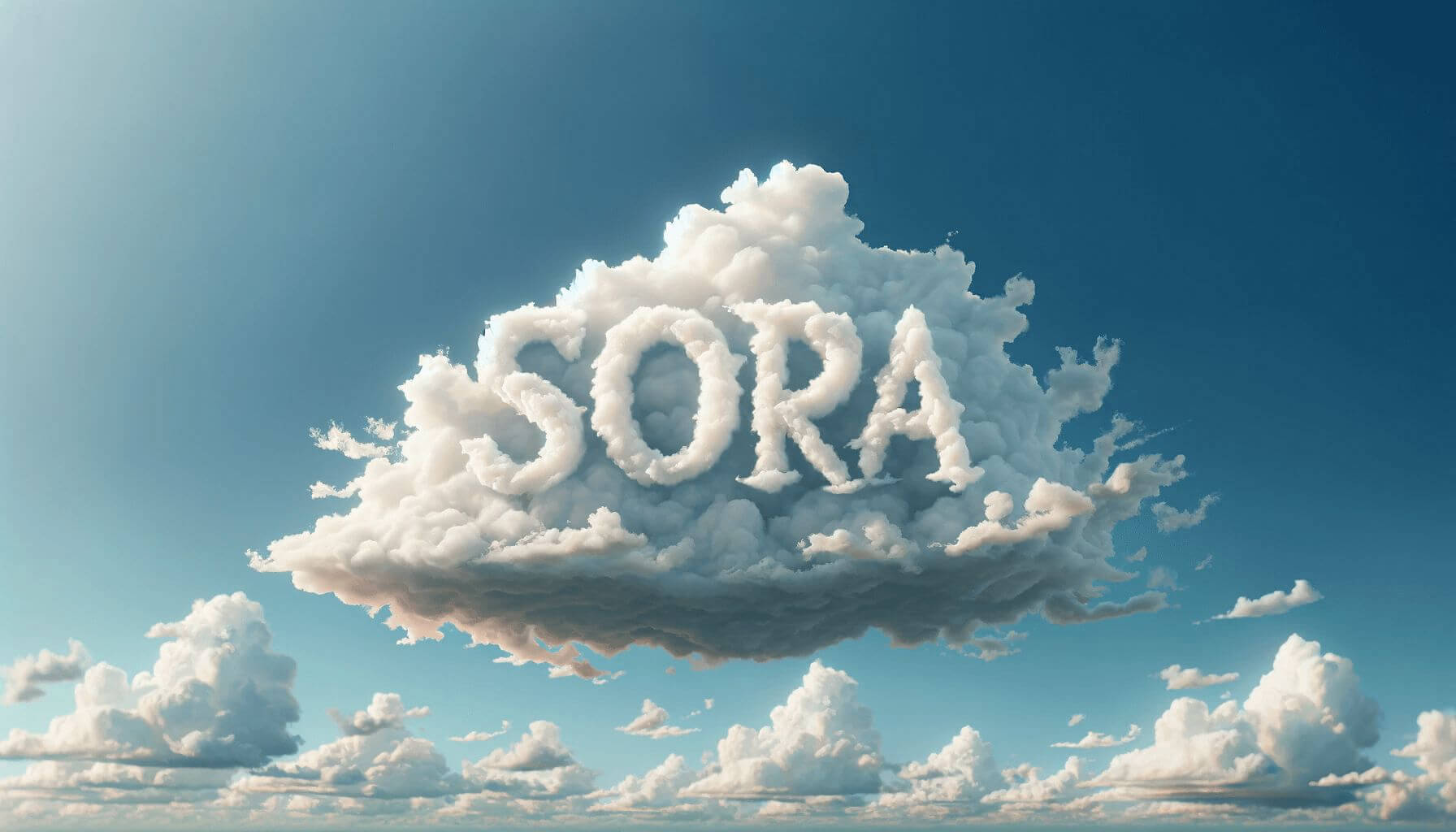
Dive into the world of Sora, OpenAI's groundbreaking AI that transforms text into captivating videos. Unleash your creativity and explore the limitless possibilities of video generation with this cutting-edge technology.
What is Sora?
Sora is a large-scale training generative model that is trained on video and image data of variable durations, resolutions, and aspect ratios. It is designed to generate high-fidelity videos up to a minute long, leveraging a transformer architecture that operates on spacetime patches of video and image latent codes.
Who developed Sora?
Sora was developed by OpenAI, as part of their exploration into large-scale training of generative models on video data.
What is the purpose of Sora?
The purpose of Sora is to serve as a generalist model of visual data, capable of generating videos and images spanning diverse durations, aspect ratios, and resolutions. It aims to be a promising path towards building general-purpose simulators of the physical world.
What are the use cases of Sora?
Sora can be used for a wide range of image and video editing tasks, such as creating perfectly looping video, animating static images, extending videos forwards or backwards in time, and transforming the styles and environments of input videos zero-shot.
Who is the target audience for Sora?
Sora is targeted towards visual artists, designers, filmmakers, and potentially other creative professionals who could benefit from its ability to generate high-quality video content based on textual prompts or pre-existing visual data.
How does Sora work?
Sora operates by first compressing videos into a lower-dimensional latent space and then decomposing the representation into spacetime patches. These patches act as transformer tokens, enabling Sora to train on videos and images of variable resolutions, durations, and aspect ratios.
What technologies are used in Sora?
Sora utilizes a diffusion model framework, combined with a transformer architecture that scales effectively for video models. It also employs techniques like video compression networks and spacetime latent patches for data representation.
How does Sora handle video and image data?
Sora turns visual data into patches by compressing it into a latent space and then decomposing it into spacetime patches. This patch-based representation allows for scalable and effective training on diverse types of videos and images.
What are Sora's notable capabilities in video generation?
Sora is capable of generating high-fidelity videos of up to a minute long, supporting various aspect ratios and resolutions. It can generate content directly at native aspect ratios for different devices and has improved framing and composition due to training on native aspect ratio videos.
How does Sora achieve long-duration video generation?
Sora's ability to generate long-duration videos is attributed to its scalable transformer architecture and diffusion model framework, which allow for effective prediction of "clean" patches from input noisy patches over time.
What range of video resolutions and aspect ratios does Sora support?
Sora supports a wide range of resolutions and aspect ratios, including widescreen 1920x1080p videos, vertical 1080x1920 videos, and everything in between, allowing for flexibility in content creation.
How does Sora handle data of varying durations and resolutions during video generation?
Sora is trained on data at its native size, without the need to resize, crop, or trim videos to a standard size. This approach provides benefits in sampling flexibility and improved framing and composition.
What unique data representation method is used during Sora's training?
During its training, Sora uses a patch-based representation for videos and images, turning them into spacetime patches that act as transformer tokens. This method is inspired by the success of tokens in large language models (LLMs) and has been found to be highly scalable and effective.
How does Sora use text prompts to generate videos?
Sora leverages highly descriptive video captions, produced through a re-captioning technique, to train on. This allows the model to improve text fidelity and overall video quality, enabling it to generate high-quality videos that accurately follow user prompts.
How does Sora ensure diversity and creativity in video generation?
Sora's generative capabilities, combined with its ability to be prompted with text, images, or videos, enable a wide range of creative outputs. Its training on internet-scale data and diverse types of visual data contribute to its generative diversity and creativity.
Can Sora edit and create based on pre-existing images or videos?
Yes, Sora can perform a wide range of image and video editing tasks based on pre-existing images or videos, including animating static images, extending videos, and transforming styles and environments of input videos zero-shot.
How does Sora achieve high-quality and detailed rendering in video generation?
Sora's high-quality and detailed rendering are achieved through its diffusion transformer architecture and the training on high-resolution images and videos. This allows for the generation of videos with dynamic camera motion and consistent movement through three-dimensional space.
Can Sora generate videos with complex environments and dynamic scenes?
Sora is capable of simulating some aspects of complex environments and dynamic scenes, including consistent 3D movement, long-range coherence, and interactions that affect the state of the world. However, it may still face challenges in accurately modeling physics and certain interactions.
What limitations or challenges does Sora face in video generation?
Sora currently exhibits limitations in accurately modeling the physics of complex interactions and may face challenges in maintaining coherence in long-duration samples or spontaneous appearances of objects. It also may struggle with certain specific instances of cause and effect.
What are the future development directions and potential applications for Sora?
The continued scaling of video models like Sora is seen as a promising path towards the development of highly capable simulators of the physical and digital world. Future developments may focus on overcoming current limitations and expanding its capabilities in simulating more complex and interactive environments.
- Authors
- Name
- Ethan Sunray
- @ethansunray